Graphcore Series E Funding: $710m Total, $440m Cash-in-Hand
by Dr. Ian Cutress on January 4, 2021 12:00 PM EST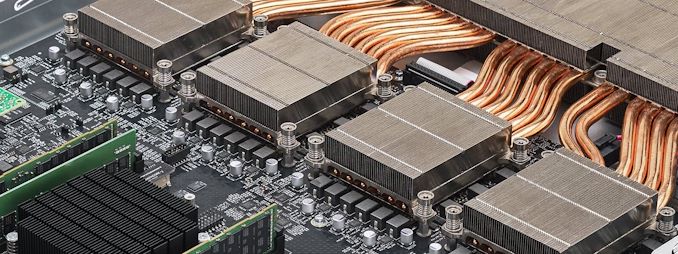
For those that aren’t following the AI industry, one of the key metrics to observe for a number of these AI semiconductor startups is the amount of funding they are able to generate. While funding is no explicit guarantee of success, it does indicate perhaps how much faith the venture capitalists (as well as OEMs and other silicon vendors) have in the technology. One of the most well-funded ventures in this space is Graphcore, and the company just announced its latest Series E funding round of $222 million, taking it to $710m total across the five rounds.
Graphcore, based in Bristol UK, is already on its second generation product, launching the Colossus MK2 GC200 in 2020. This chip contains 60 billion transistors, 900MB of built-in memory, is manufactured on TSMC’s N7 node at 823 mm2, and can achieve 250 TFLOPs of AI compute. Graphcore bundles four of them into a 1U chassis along with an Arm-based control chip and a crazy amount of networking to enable a network containing up to 64000 chips. Customers can order this IPU-M2000 unit, or 16 of them in a dedicated rack. Graphcore also provides the POPLAR software stack, with direct support for PyTorch, TensorFlow, ONNX, and PaddlePaddle machine learning frameworks.
The latest $222m Series E funding round was led by Ontario Teachers’ Pensions Plan Board (what?), with additional funds managed by Fidelity International and Schroders as new investors. Previous investors also participated, including Baillie Gifford and Draper Esprit. With the latest round of funding bringing the total up to $710m, this would put Graphcore at #2 in terms of AI Chip pure-play startups. This is just behind the $850m invested into Chinese semiconductor startup Horizon Robotics, founded by a CEO Yu Kai a Baidu veteran, of which the latest $150m round finished in December. SambaNova is #3 with $456m, and Nuvia has $293m. The latest round of funding brings Graphcore’s valuation to $2.77 billion.
With Graphcore’s first generation product, the company aligned with Dell to provide server units featuring eight add-in PCIe cards, each with two of its first generation IPUs. The company is claiming that the newest second generation MK2 is rolling out to more customers even during COVID times, especially to academic research such as UMass, Oxford, and ICL. Official details on its corporate customers seem somewhat thin, beyond an official tie-in with Microsoft, however Graphcore has said that they are currently working with hyperscalers and financial service companies.
This is perhaps why Graphcore also stating that it has $440m cash-in-hand is quite important. As every startup has an effective burn rate of capital, this should be sufficient for the company to also go out to enable more customers, as well as develop next generation products. Graphcore has already announced through TSMC that it is already scoping TSMC’s 3nm process for a future product line. Graphcore is also a member of the recently formed MLCommons, the governing body behind MLPerf, and expects to participate with its first submissions on MK2 in Q2 this year.
Source: Graphcore
Related Reading
- TSMC and Graphcore Prepare for AI Acceleration on 3nm
- SambaNova Breaks Cover: $450M AI Startup with 8-Socket AI
- NUVIA Completes Series B Funding Round: $240M
- 342 Transistors for Every Person In the World: Cerebras 2nd Gen Wafer Scale Engine Teased
- NVIDIA Announces A100 80GB: Ampere Gets HBM2E Memory Upgrade
12 Comments
View All Comments
jtd871 - Monday, January 4, 2021 - link
Public employee (Ontario, Canada teachers in this case) pension funds are big institutional investors.ksec - Monday, January 4, 2021 - link
There are very limited information on Graph Core. How are they any different to other Machine Learning processors like TPU from Google or NPU within an Apple SoC.Will there be an in-depth article, or even a high level overview on Graphcore?
Yojimbo - Monday, January 4, 2021 - link
It's very different from the NPU within an Apple SoC for a couple of reasons. Firstly those neural coprocessors on SoCs are generally CNN inference ASICs. CNN inference is simpler than training and something like Graphcore's chip is designed to accelerate more than just CNNs (in inference and in training). Secondly, Graphcore has built a scalable systems-level architecture, not just a chip that can be integrated in an SoC. It's (comparatively) much easier to build something like an NPU and integrate it into an SoC than it is to design a scale-out architecture.As far as the TPU, it's similar but there are differences in the implementation. You can go to www.nextplatform.com if you want to see some information on the TPU, graphcore, or other ai accelerators.
galeos - Monday, January 4, 2021 - link
Citadel have got an interesting paper on arXiv where there attempt to analyse the Graphcore architecture via microbenchmarking. Worth a look: https://arxiv.org/abs/1912.03413JohnLeonard - Tuesday, January 5, 2021 - link
Hi ksec, these resources may help give some insight into what Graphcore have done and how it differs in approach to the existing approaches to ML/AI workloads.https://www.graphcore.ai/resources/white-papers
Feel free to reach out if you need any further information.
thanks,
John Leonard- Product Marketing Manager, Graphcore.
ksec - Wednesday, January 6, 2021 - link
Thanks.Yojimbo - Monday, January 4, 2021 - link
Funding secured is often more an indication of how much hype there is for the people/product involved and how good of salesmen the people are. Take a look at MagicLeap and Theranos.Yojimbo - Monday, January 4, 2021 - link
Regarding $440m cash on hand. I wonder if that's really enough to design and produce a 3 nm chip.TomWomack - Monday, January 4, 2021 - link
Remember it's very much a step-and-repeat sort of chip - version 1 was 19*2^6 copies of a block comprising a core with a complete-16-FP-multiplies-per-cycle unit and 256kb of RAM, this version 2 is probably a larger number of copies of a very similar block. If you were asked to pick something that's not too hard to port to a new smaller process, you'd pick this.Calin - Tuesday, January 5, 2021 - link
Well, $440M is small change in the fab industry. And to produce a chip on 3 nm, Intel would give ten times that (as it probably already gave for the 10nm technology to small effect).